Human-AI Teaming During an Ongoing Disaster
BY TARA TASUJI & KERI K. STEPHENS, THE UNIVERSITY OF TEXAS AT AUSTIN | JUL 22, 2023
Bringing research into the real world… of baseball
In 2023, CBS Austin, Texas reported on a system being tested during the Round Rock Express baseball games that automates the calling of balls and strikes. Nicknamed the “robot umpire,” the elaborate camera system makes the call and relays the information to the human umpire.
While I do not follow baseball regularly, I’ve watched this practice unfold with great interest: it is a real-world example of automating a process where human experts—umpires—are being asked to team with machines to make decisions. We see this in many aspects of our lives, and quite often it happens behind the scenes. But we are only now beginning to understand how the shared decisions are actually working and impacting the humans involved in the process.
The following paper, published in Human Machine Communication in July of 2023, isn’t about baseball, but it does make me wonder if the human umpires ever question the calls of the robot umpire, or if they accept them without doubt.
– Keri K. Stephens, July 2023
Summary
A new study by a team of researchers from the Moody College of Communication at University of Texas at Austin’s Technology and Information Policy Institute, Brigham Young University, George Mason University, and Virginia Tech found that when people train and give feedback to a machine involved in machine learning/artificial intelligence, sometimes they communicate with the machine as if it is a valued teammate.
Specifically, when people are asked to correct a machine’s mistakes, they express self-doubt and uncertainty when they realize they disagree with a machine’s decision. Some people discuss teaching the machine over time much the same way as they would teach and correct a puppy’s behavior—showing delight when the machine performs well and disappointment when the machine is not learning as quickly as they had hoped.
This display of complex emotions indicates that some humans come to view the machine as a partner working with them towards an overarching goal.
Problem
During a disaster, humans can work with Artificial Intelligence (AI)-infused systems (i.e., machines) to find and label crisis-relevant information by sifting through large quantities of data posted on social media platforms.1,2
In addition to humans labeling social media data for the machine to learn how to detect patterns, they also “grade” and correct the machine to steer the machine’s decision-making in the right direction.3
When people train and correct a machine, they often rely on scripts—or the collection of facts that people use to know what behaviors are appropriate in a given situation.4
Understanding the experiences and feelings of people who engage in this form of machine-related work can ultimately help emergency responders support their teams during disaster events.
Project Co-PI, Amanda Hughes, interviews a person as they label Tweets to train the machine
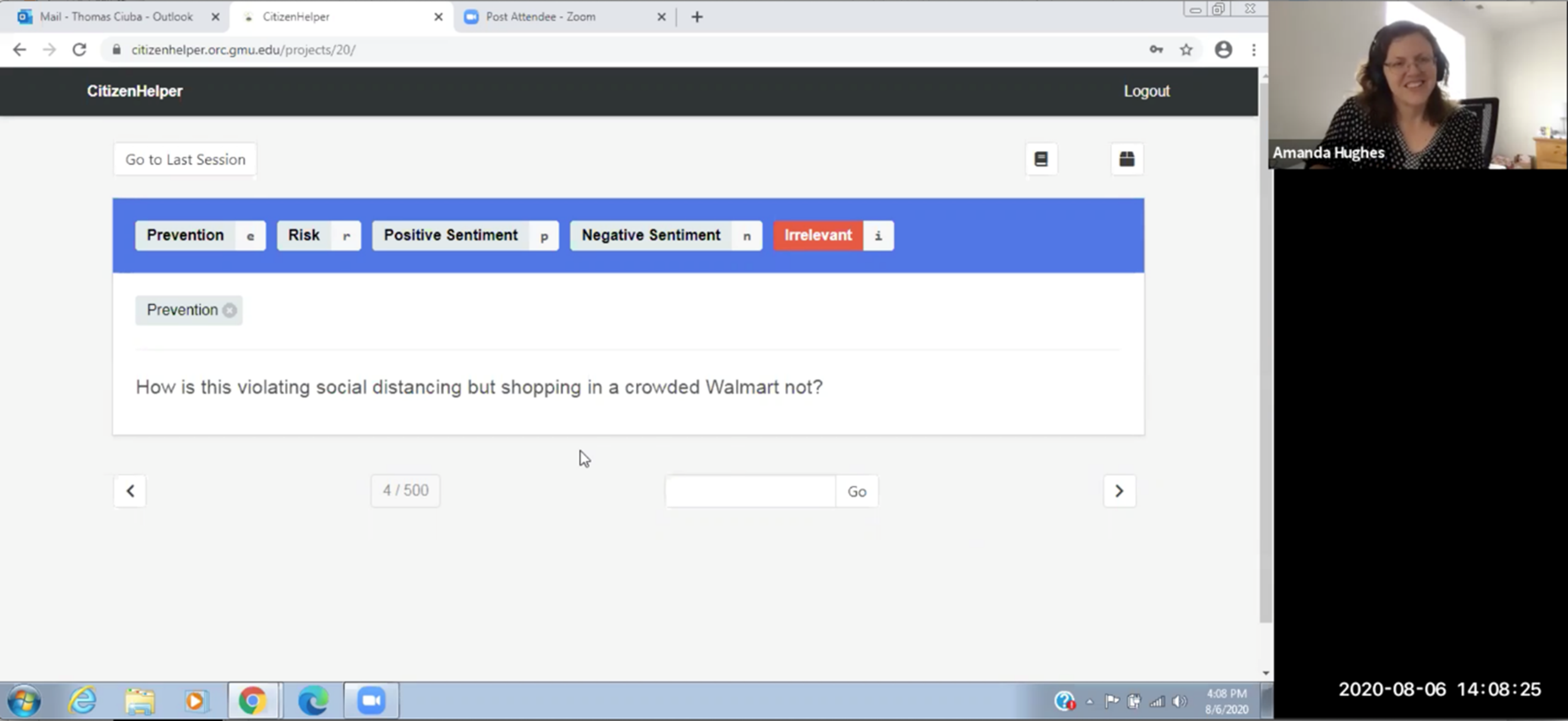
Key Takeaways
Study findings reveal that people—whether they are labeling tweets for the machine to learn or verifying/correcting the machine’s labels—rely on scripts to cope with the challenges they face when making decisions about tweets. Coping strategies include regularly referring to their training or reminding themselves about the goals of the project. Interestingly, people experience greater difficulty and self-doubt—and relatively fewer coping strategies—when they find themselves disagreeing with the machine’s judgment and ultimately deciding to correct the machine’s labels. This finding suggests that people are rather reluctant to second-guess a machine’s performance.
People also rely on scripts that influence their ability to label and verify/correct tweets—such as the background or expertise they bring to the tasks as well as their desire to learn, or how they view the machine. We found that when people are checking and correcting the machine’s labels, they are more focused on the machine’s learning—showing frustration when the machine is not learning and excitement when the machine is improving—and even associate the machine’s learning process with how an animal or person learns. This is what we refer to as behavioral anthropomorphism.
People involved in helping machines learn engage in different degrees of behavioral anthropomorphism: some individuals view the machine as only a tool, while others consider the machine to be a very helpful and useful partner or teammate. People also score higher on behavioral anthropomorphism the longer they work to train a machine. This finding suggests that people may be applying the same scripts they would use to teach a toddler or puppy to a machine because they believe the machine also aspires to learn new skills and information. People are then willing to treat the machine with patience and understanding as it takes its “first stumbling steps” towards proficiency.
The NSF CIVIC Project Team (including our CIVIC Partners)
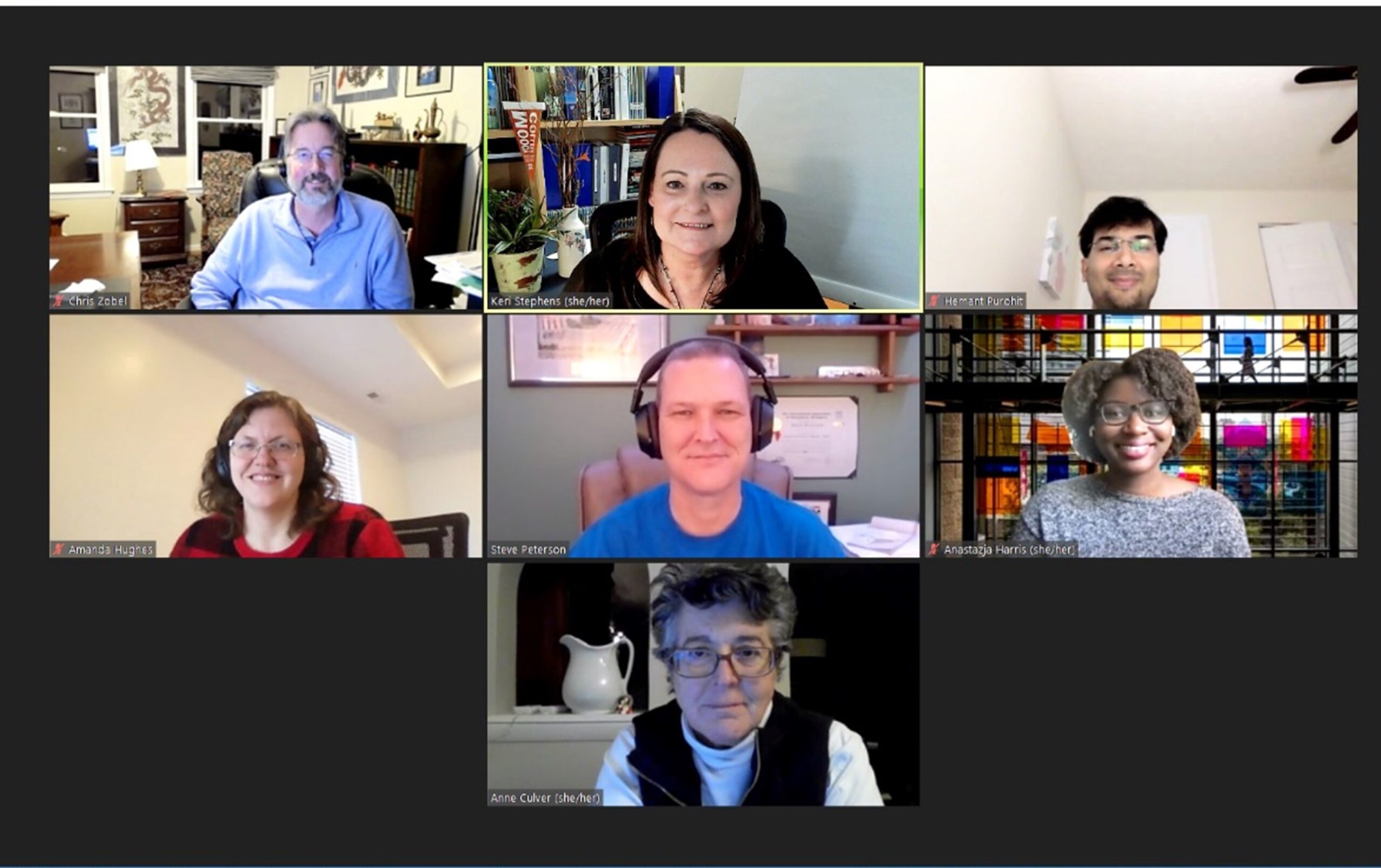
Methodology
Our research team used an AI-infused system called CitizenHelper5 to examine tweets and find valuable information for emergency responders during the COVID-19 pandemic. For example, emergency responders wanted to know if workers needed additional support at emergency supply distribution centers to maintain social distancing. Over two rounds of data collection, we conducted 55 interviews over Zoom with 14 Community Emergency Response Team (CERT)6 participants. During the first round of data collection (in May and June 2020), each CERT volunteer was asked to train CitizenHelper by labeling 500 tweets that were randomly selected from a dataset collected via the Twitter stream.7 During this labeling task, volunteers could refer to a detailed coding book with examples of each label. The labels were created in consultation with the CERT leader, and they were deemed important for emergency managers. Volunteers assigned the following labels to each tweet:
-
- Relevant: Tweets containing COVID-19-related activity in the Washington, D.C. Metro region. Relevant tweets were then considered for one or more additional labels: Prevention, Risk, Positive sentiment, and/or Negative sentiment.
-
- Prevention: Tweets containing information about how people were preventing the spread of COVID-19.
- Risk: Tweets containing information about risky behaviors related to COVID-19.
- Positive sentiment: Tweets containing views around positive COVID-19 actions.
- Negative sentiment: Tweets containing views around negative COVID-19 actions.
-
- Relevant: Tweets containing COVID-19-related activity in the Washington, D.C. Metro region. Relevant tweets were then considered for one or more additional labels: Prevention, Risk, Positive sentiment, and/or Negative sentiment.
During the second round of data collection (in July and early August 2020), CERT volunteers were provided with 250 tweets that had already been labeled by CitizenHelper, and they were asked to verify or correct the machine’s labels.8 It was during the correction process that people expressed self-doubt concerning their own opinions and expertise: they often wondered if the machine knew more than they did, and thus, they were reluctant to correct the machine.
Acknowledgement:
We want to thank our incredible community partner, Steve Peterson, and the Montgomery County CERT volunteers who allowed us to interview them as part of this project.
This project was funded by a National Science Foundation Award # 2029692, RAPID/Collaborative Research: Human-AI Teaming for Big Data Analytics to Enhance Response to the COVID-19 Pandemic; and Award #2043522, SCC-CIVIC-PG Track B: Assessing the Feasibility of Systematizing Human-AI Teaming to Improve Community Resilience.
Any opinions, findings, and conclusions or recommendations expressed in this material are those of the authors and do not necessarily reflect the views of the National Science Foundation.
References and Additional Information
1. Imran, M., Castillo, C., Diaz, F., & Vieweg, S. (2015). Processing social media messages in mass emergency: A survey. ACM Computing Surveys, 47(4), 67:1–67:38. https://doi.org/10.1145/2771588
2. Purohit, H., Castillo, C. Imran, M., & Pandey, R. (2018). Social-EOC: Serviceability model to rank social media requests for emergency operation centers. 2018 IEEE/ACM International Conference on Advances in Social Networks Analysis and Mining (ASONAM), 119–126. https://doi.org/10.1109/ASONAM.2018.8508709
3. Amershi, S., Cakmak, M., Know, W.B., & Kulesza, T. (2014). Power to the people: The role of humans in interactive machine learning. AI Magazine, 35(4), 105–120. https://doi.org/10.1609/aimag.v35i4.2513
4. Gioia, D. A., & Poole, P. P. (1984). Scripts in organizational behavior. The Academy of Management Review, 9(3), 449–459. https://doi.org/10.2307/258285
5. Pandey, R., & Purohit, H. (2018, August). Citizenhelper-adaptive: Expert-augmented streaming analytics system for emergency services and humanitarian organizations. In 2018 IEEE/ACM international conference on advances in social networks analysis and mining (ASONAM) (pp. 630-633). IEEE. https://doi.org/10.1609/icwsm.v11i1.14863
6. Community Emergency Response Team (CERT) volunteers are taught about disaster preparedness and disaster response skills (e.g., light search and rescue, fire safety, disaster medical operations). See FEMA (2022) for more information.
7. During the first phase of data collection, our research team conducted a total of 41 interviews: 13 CERT volunteers were interviewed and observed on three separate occasions, and the CERT leader was interviewed on two separate occasions. Using the Twitter Streaming Application Programming Interface (API) and its geo-fencing method, our team of researchers filtered 2.1 million tweets originating from the Washington, D.C. Metro region between March and May 2020. Based on a curated list of 1,521 CERT-approved keywords, we then screened potentially relevant tweets for COVID-19 response. A total of 14,000 tweets were randomly selected from the resulting filtered tweets to give us the dataset for the CERT volunteers.
8. During the second phase of data collection, our research team conducted a total of 14 interviews: 7 of the phase I CERT volunteers were interviewed and observed on two separate occasions.